What Are the Best Methods for Forecasting Demand Accurately?
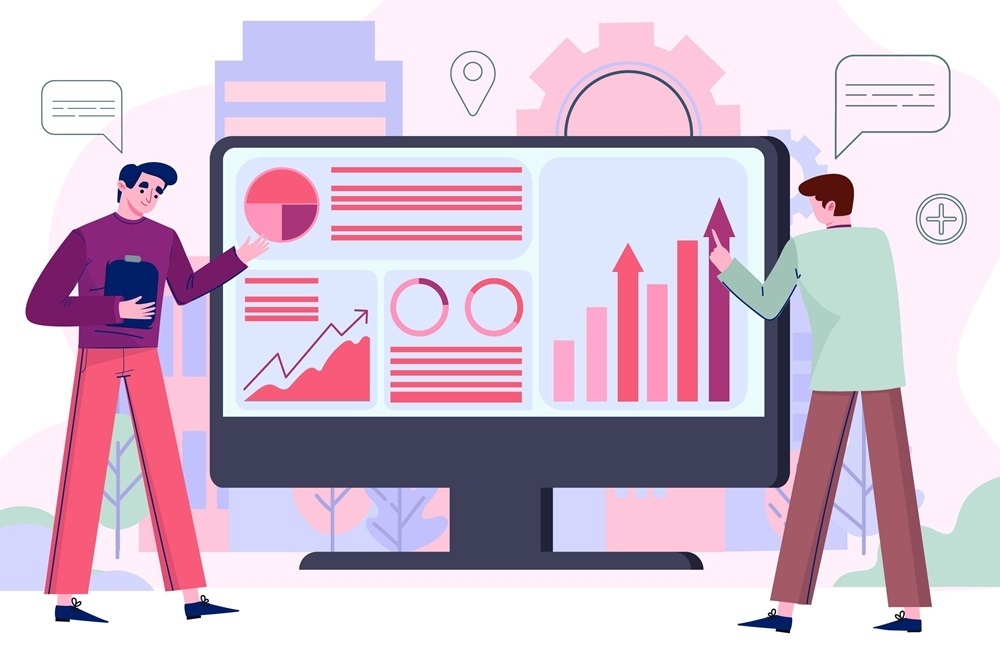
Forecasting demand is a critical aspect of business planning and operations. Forecasting demand methods allow businesses to optimize inventory levels, streamline operations, and improve customer satisfaction. In this blog, we will explore the best methods for forecasting demand accurately, focusing on the types of demand forecasting and how these methods can be effectively applied.
Importance of Demand Forecasting
Demand forecasting is the process of predicting future customer demand for a product or service. Accurate demand forecasting is essential for businesses to plan production, manage inventory, and allocate resources efficiently. It helps businesses minimize costs, reduce waste, and ensure that they meet customer needs without overproducing.
Types of Demand Forecasting
There are several types of demand forecasting, each suited for different purposes and time horizons. Understanding these types of demand forecasting is crucial for selecting the appropriate forecasting demand methods.
Qualitative Forecasting:
This type relies on expert judgment and market research. It is often used when historical data is not available or when launching new products. Techniques include market surveys, the Delphi method, and expert panels.
Quantitative Forecasting:
This type uses historical data to predict future demand. It includes methods like time series analysis, causal models, and regression analysis. Quantitative forecasting is typically more accurate for short- to medium-term predictions.
Short-term Forecasting:
This focuses on predicting demand for a few days to a few months. It is essential for managing daily operations and inventory levels.
Medium-term Forecasting:
This covers a period of a few months to a couple of years. It is used for planning production schedules, workforce requirements, and budget allocations.
Long-term Forecasting:
This type looks several years into the future. It is crucial for strategic planning, capacity expansion, and long-term investment decisions.
Best Forecasting Demand Methods
Selecting the right forecasting demand methods depends on the type of demand forecasting you are conducting. Here are some of the best forecasting demand methods:
1. Time Series Analysis
Time series analysis is a popular quantitative forecasting demand method. It involves checking the previous data to identify patterns and trends. This method is particularly useful for short- and medium-term forecasting. Common techniques include:
Moving Averages: This technique smooths out short-term fluctuations and highlights longer-term trends by averaging data points over a specified period.
Exponential Smoothing: This forecasting demand method gives more weight to recent observations, making it more responsive to changes in the data.
ARIMA (AutoRegressive Integrated Moving Average): ARIMA models are sophisticated tools that can handle different types of time series data, including seasonal variations.
2. Causal Models
Causal models are used when there is a known relationship between the demand and one or more independent variables. This method is effective for medium- to long-term forecasting. Techniques include:
Regression Analysis: This statistical method analyzes the relationship between demand and factors such as price, advertising spending, and economic indicators.
Econometric Models: These models incorporate economic theories and data to forecast demand based on variables like GDP growth, interest rates, and consumer confidence.
3. Qualitative Methods
When historical data is limited or the market environment is highly uncertain, qualitative methods can be valuable. These methods rely on expert judgment and insights. Common qualitative techniques include:
Delphi Method: This iterative process gathers opinions from a panel of experts, refining forecasts through multiple rounds of questioning.
Market Research: Surveys, focus groups, and interviews with customers and industry experts provide valuable insights into future demand trends.
Scenario Planning: This method explores different future scenarios and their potential impact on demand, helping businesses prepare for various contingencies.
4. Machine Learning and Artificial Intelligence
Advancements in technology have introduced sophisticated forecasting demand methods using machine learning and AI. These methods can analyze vast amounts of data and identify complex patterns that traditional methods might miss. Techniques include:
Neural Networks: These algorithms mimic the human brain's structure and are capable of learning from data to make accurate predictions.
Support Vector Machines: SVMs are powerful tools for classification and regression tasks, making them suitable for demand forecasting.
Integrating Forecasting Methods
Often, the best approach to forecasting demand involves combining multiple methods to leverage their strengths and mitigate their weaknesses. This integrated approach, known as a hybrid model, can provide more accurate and reliable forecasts.
For example, a business might use time series analysis for short-term forecasting while employing causal models for medium-term predictions. Machine learning algorithms can be integrated to refine these forecasts further, especially in rapidly changing environments.
Best Practices for Accurate Demand Forecasting
Data Quality:
Ensure that the data used for forecasting is accurate, complete, and up-to-date. Poor data quality can lead to incorrect forecasts and misguided decisions.
Continuous Monitoring:
Regularly review and update forecasts to reflect changes in market conditions, customer behavior, and other relevant factors.
Collaboration:
Involve stakeholders from different departments, such as sales, marketing, and finance, to provide a comprehensive view of demand drivers.
Scenario Analysis:
Prepare for various scenarios by exploring different assumptions and their potential impact on demand.
Technology Utilization:
Use advanced forecasting tools and software to increase reliability and effectiveness.
Challenges in Demand Forecasting
Despite the availability of advanced forecasting demand methods, demand forecasting accurately remains challenging due to several factors:
Market Volatility:
Rapid changes in market conditions, consumer preferences, and economic factors can make accurate forecasting difficult.
Data Limitations:
Incomplete or inaccurate data can compromise the quality of forecasts.
Bias and Subjectivity:
Qualitative methods are prone to biases, and even quantitative methods can be influenced by the assumptions made by analysts.
Technological Complexity:
Implementing and maintaining sophisticated forecasting models requires specialized skills and resources.
Conclusion
Accurate demand forecasting is a vital component of successful business planning. By understanding the types of demand forecasting and selecting the appropriate methods, businesses can improve their ability to predict future demand and make informed decisions. Whether using time series analysis, causal models, qualitative methods, or advanced machine learning techniques, the key is to integrate multiple approaches and continuously refine forecasts based on the latest data and market insights.
In summary, the best methods for forecasting demand accurately involve a combination of qualitative and quantitative techniques, supported by advanced technology and continuous monitoring. By following best practices and addressing the inherent challenges, businesses can achieve more reliable demand forecasts, leading to better resource allocation, improved customer satisfaction, and enhanced overall performance.
FAQs
1. What is the best method for short-term demand forecasting?
For short-term demand forecasting, time series analysis methods such as moving averages and exponential smoothing are highly effective. These methods analyze historical data to identify patterns and trends that can be used to predict near-term demand.
2. How can machine learning improve demand forecasting accuracy?
Machine learning improves demand forecasting accuracy by analyzing large datasets and identifying complex patterns that traditional methods may miss. Techniques like neural networks and random forests can provide more precise predictions by continuously learning from new data.
3. What are the main challenges in demand forecasting?
The main challenges in demand forecasting include market volatility, data limitations, and the complexity of implementing advanced models. Additionally, biases in qualitative methods and the need for specialized skills to manage sophisticated forecasting tools can also pose significant hurdles.
Predict your sales volume and demand trends with our Artificial Intelligence-based SaaS platform visit: https://thousense.ai/
- Industry
- Art
- Causes
- Crafts
- Dance
- Drinks
- Film
- Fitness
- Food
- Games
- Gardening
- Health
- Home
- Literature
- Music
- Networking
- Other
- Party
- Religion
- Shopping
- Sports
- Theater
- Wellness
- News