Unleashing Federated Learning: An Introduction to Decentralized Intelligence
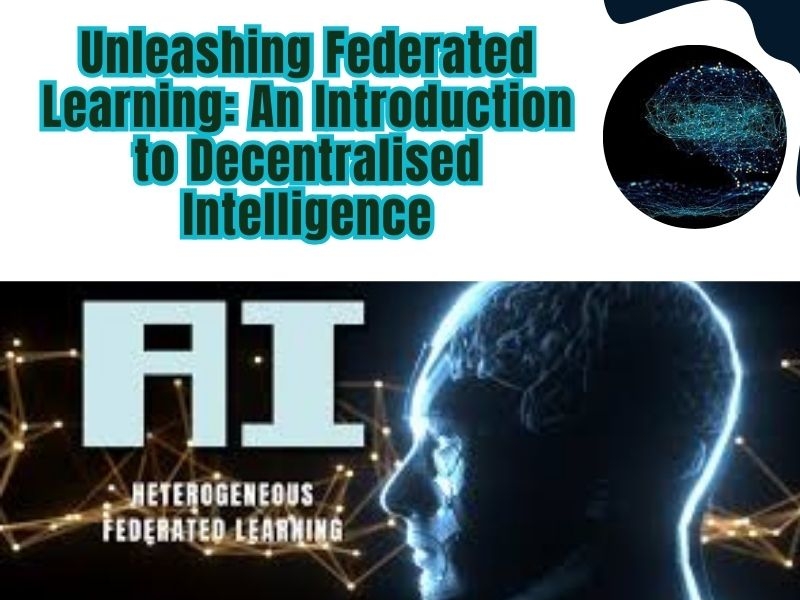
Federated learning represents a groundbreaking approach to machine learning that is revolutionizing how models are trained and deployed across distributed devices and networks. Unlike traditional centralized machine learning, where data is aggregated into a central server for training, federated learning enables decentralized intelligence by training models directly on edge devices while preserving data privacy and security. This innovative paradigm is reshaping the landscape of machine learning and unlocking new opportunities for collaborative learning, personalization, and privacy preservation. In this article, we'll delve into the concept of federated learning, explore its key principles, applications, and benefits, and discuss its implications for the future of AI.
Understanding Federated Learning:
Federated learning is a decentralized machine learning approach that enables training models across a network of distributed devices, such as smartphones, IoT devices, or edge servers, without sharing raw data. Instead of sending data to a central server for training, federated learning leverages local computation and communication to train models directly on edge devices while aggregating model updates to improve overall performance. This distributed learning paradigm allows devices to collaboratively learn from decentralized data sources while preserving privacy, security, and data ownership.
Key Principles of Federated Learning:
Decentralized Training:
Federated learning distributes model training across edge devices, enabling local computation and model updates without centralizing. if you want to learn more about Data Science, we can help you by applying from here best data science course
Privacy Preservation:
Federated learning ensures data privacy by keeping raw data on local devices and only sharing model updates with a central server or aggregator.
Collaborative Learning:
Federated learning facilitates collaborative learning by aggregating model updates from multiple devices to improve model performance across the entire network.
Edge Computing:
Federated learning leverages edge computing infrastructure to perform local model training and inference, reducing latency and bandwidth requirements.
Adaptive Learning:
Federated learning enables adaptive learning by incorporating feedback and updates from edge devices in real-time, allowing models to continuously improve and adapt to changing data distributions.
Applications of Federated Learning:
Mobile Devices:
Federated learning is widely used in mobile applications to train personalized models directly on users' smartphones while preserving privacy.
IoT Devices:
Federated learning enables IoT devices, such as sensors and smart appliances, to collaboratively learn from decentralized data sources while minimizing communication overhead.
Healthcare:
Federated learning is applied in healthcare settings to train predictive models on patients' health data distributed across hospitals, clinics, and wearable devices while ensuring patient privacy and compliance with regulations.
Finance:
Federated learning is utilized in the finance industry to train fraud detection models directly on banking customers' devices while protecting sensitive financial data.
Smart Cities:
Federated learning is deployed in smart city initiatives to analyze data from distributed sensors and devices for traffic management, environmental monitoring, and urban planning.
Benefits of Federated Learning:
Privacy Preservation:
Federated learning protects user privacy by keeping sensitive data on local devices and only sharing model updates.
Data Security:
Federated learning enhances data security by reducing the risk of data breaches and unauthorized access to sensitive information.
Scalability:
Federated learning scales efficiently to large datasets and diverse device types, enabling collaborative learning across decentralized networks.
Personalization:
Federated learning enables personalized model training on individual devices, leading to tailored recommendations and user experiences.
Resource Efficiency:
Federated learning minimizes communication overhead and bandwidth usage by performing model training locally on edge devices.
Future Outlook:
Federated learning represents a promising approach to decentralized intelligence that addresses the challenges of privacy, security, and scalability in machine learning. As the adoption of edge computing and IoT devices continues to grow, federated learning is poised to play a pivotal role in enabling collaborative and adaptive learning across distributed networks. With ongoing research and advancements in federated learning techniques, we can expect to see broader adoption and innovative applications in various domains, driving the next wave of decentralized intelligence and AI innovation. best data science course with placement guarantee
conclusion,
Federated learning offers a compelling solution to the challenges of centralized machine learning, enabling decentralized intelligence, privacy preservation, and collaborative learning across distributed networks. As organizations increasingly embrace edge computing and IoT technologies, federated learning is set to revolutionize how models are trained, deployed, and utilized in the era of decentralized intelligence.
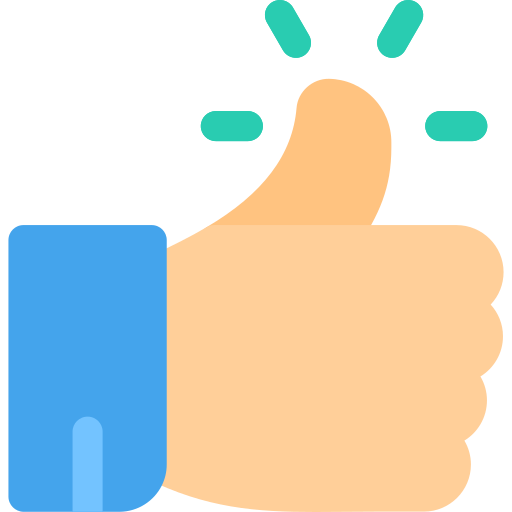
- Industry
- Art
- Causes
- Crafts
- Dance
- Drinks
- Film
- Fitness
- Food
- الألعاب
- Gardening
- Health
- الرئيسية
- Literature
- Music
- Networking
- أخرى
- Party
- Religion
- Shopping
- Sports
- Theater
- Wellness
- News