"AI & Money: The Power of Machine Learning in Finance"
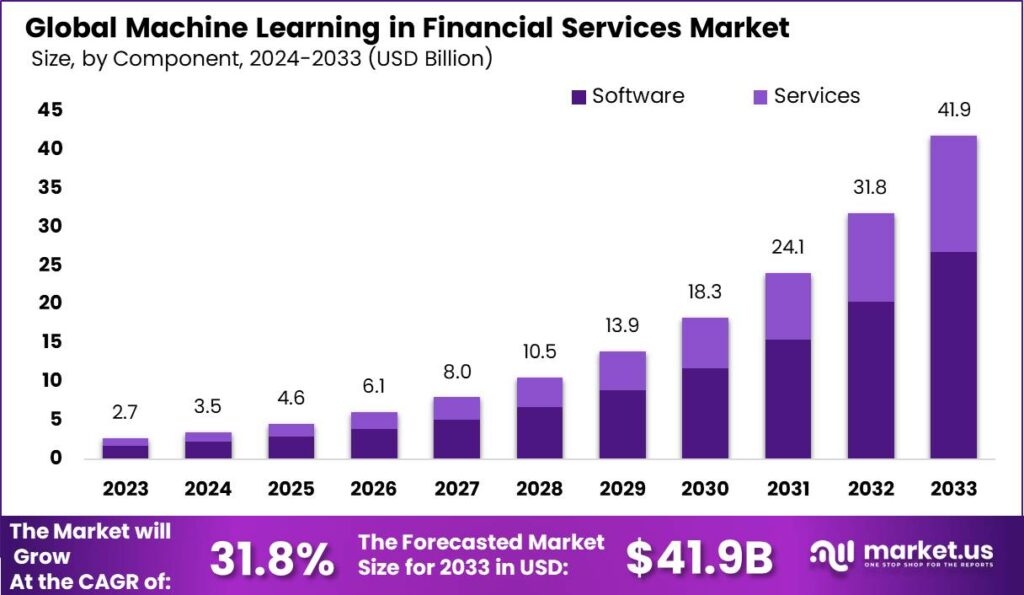
Introduction
Machine learning (ML) is rapidly transforming the financial services industry, offering a range of applications that enhance decision-making, reduce risks, and improve customer experiences.
Read More - https://market.us/report/machine-learning-in-the-financial-services-market/
Growth in this market is driven by the increasing availability of big data, advancements in computing power, and the financial industry's need for more sophisticated analytical tools. The adoption of ML allows financial institutions to analyze large volumes of data in real-time, leading to more accurate predictions and better customer service. However, challenges such as data privacy concerns, the need for skilled talent, and the complexity of integrating ML into existing systems remain significant barriers. For new entrants, the opportunities are vast, particularly in areas where traditional financial institutions are slow to adapt. By leveraging innovative ML solutions, startups can carve out niche markets, offering specialized services that address specific industry needs.
Emerging Trends
-
AI-Powered Chatbots and Virtual Assistants: Financial institutions are increasingly using AI-driven chatbots to enhance customer service, providing quick, personalized responses and streamlining operations.
-
Fraud Detection and Prevention: ML algorithms are becoming essential in identifying fraudulent activities, enabling real-time detection and reducing the financial impact of fraud.
-
Algorithmic Trading: The use of ML in trading strategies is gaining traction, with algorithms that can process vast amounts of data and execute trades faster than human traders.
-
Credit Scoring and Risk Management: Traditional credit scoring models are being replaced by ML models that consider a wider range of data points, offering more accurate assessments of creditworthiness.
-
Personalized Financial Services: ML is enabling the creation of highly personalized financial products, tailored to individual customer needs and preferences.
Top Use Cases
-
Risk Management: ML models are used to predict and mitigate risks, including credit, market, and operational risks, by analyzing historical data and identifying potential future risks.
-
Fraud Detection: Financial institutions use ML to detect and prevent fraudulent transactions by analyzing patterns and anomalies in real-time.
-
Customer Relationship Management (CRM): ML helps in analyzing customer data to provide personalized financial products and services, improving customer satisfaction and loyalty.
-
Portfolio Management: ML algorithms assist in optimizing investment portfolios by analyzing market trends and predicting asset performance.
-
Regulatory Compliance: ML tools are used to ensure compliance with financial regulations by monitoring transactions and flagging suspicious activities.
Major Challenges
-
Data Privacy Concerns: Ensuring the privacy and security of sensitive financial data is a significant challenge, especially with increasing cyber threats.
-
Regulatory Compliance: Navigating the complex and evolving regulatory landscape is difficult, as ML algorithms must comply with stringent financial regulations.
-
Integration with Legacy Systems: Many financial institutions struggle to integrate ML technologies with their existing systems, which can be outdated and inflexible.
-
Lack of Skilled Talent: There is a shortage of professionals with the expertise needed to develop, implement, and manage ML systems in the financial sector.
-
Ethical Considerations: The use of ML in finance raises ethical questions, particularly around fairness, transparency, and accountability.
Market Opportunity
-
Emerging Markets: The adoption of ML in financial services is growing rapidly in emerging markets, where there is less reliance on legacy systems, offering significant opportunities for new entrants.
-
Niche Applications: Startups can focus on niche areas within the financial sector, such as micro-lending, where ML can provide tailored solutions that large institutions may overlook.
-
RegTech Solutions: There is a growing demand for regulatory technology (RegTech) solutions that use ML to help financial institutions comply with complex regulations efficiently.
-
Collaborative Opportunities: New entrants can collaborate with established financial institutions to develop and implement ML solutions, leveraging their resources and customer base.
-
Financial Inclusion: ML can play a critical role in increasing financial inclusion by offering tailored financial products to underserved populations.
Conclusion
Machine learning is revolutionizing the financial services industry by offering tools that enhance decision-making, improve customer service, and reduce risks. While the market offers significant growth opportunities, particularly for new entrants, challenges such as data privacy, regulatory compliance, and integration with legacy systems must be addressed.
Emerging trends like AI-powered chatbots, personalized financial services, and fraud detection highlight the transformative potential of ML in finance. Despite the challenges, the opportunities for innovation and growth in this market are immense, with the potential to drive significant changes in how financial services are delivered and consumed.
- Industry
- Art
- Causes
- Crafts
- Dance
- Drinks
- Film
- Fitness
- Food
- Игры
- Gardening
- Health
- Главная
- Literature
- Music
- Networking
- Другое
- Party
- Religion
- Shopping
- Sports
- Theater
- Wellness
- News