Explainable Perception in Robotics: Clarifying Decision-Making in Sensory Processing
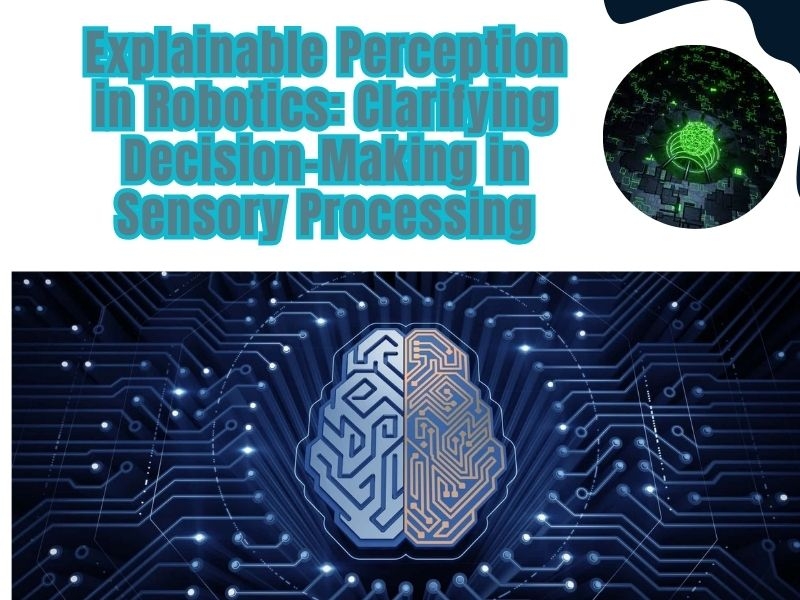
Explainable perception in robotics refers to the ability to provide clear explanations for the decision-making processes underlying sensory processing in robotic systems. It aims to enhance transparency, trust, and interpretability in the interactions between robots and humans by making the reasoning behind robotic actions understandable and interpretable. If you are looking forward to making your future in Robotics, here are top institutes for data science course. Here's how explainable perception works and its significance in robotics:
Transparent Decision-Making:
Explainable perception involves designing robotic systems that can articulate the reasoning behind their sensory processing and decision-making processes in a transparent and understandable manner. This transparency enables humans to comprehend why a robot made a particular decision or took a specific action.
Interpretable Models and Representations:
Explainable perception relies on interpretable models and representations that capture the internal mechanisms of the robotic system's sensory processing algorithms. These models should be designed to be easily interpretable by humans, allowing them to understand how the robot perceives and interprets sensory information.
Feature Visualization and Explanation:
Techniques such as feature visualization and explanation methods are used to provide insights into the features or patterns in sensory data that influence the robot's decision-making process. By visualizing and explaining these features, humans can gain a deeper understanding of how the robot perceives its environment and makes decisions based on sensory inputs.
Contextual Understanding:
Explainable perception considers the context in which robotic decisions are made, taking into account factors such as the robot's goals, constraints, and environmental conditions. By providing contextual explanations for robotic actions, humans can better understand the rationale behind the robot's behavior and anticipate its actions in different situations.
Human-Robot Collaboration and Trust:
Explainable perception fosters collaboration and trust between humans and robots by promoting transparency and comprehension in their interactions. When humans can understand and interpret the reasoning behind robotic decisions, they are more likely to trust the robot's capabilities and collaborate effectively with it in various tasks and scenarios.
Safety and Reliability:
In safety-critical applications such as autonomous vehicles or robotic surgery, explainable perception is essential for ensuring the safety and reliability of robotic systems. By providing clear explanations for their actions, robots can help mitigate the risks associated with unexpected behavior or errors in sensory processing.
Ethical and Social Implications:
Explainable perception also raises important ethical and social implications related to the accountability, responsibility, and transparency of robotic systems. By making robotic decision-making processes understandable and interpretable, we can address concerns about bias, fairness, and unintended consequences in AI-driven systems.
Conclusion
explainable perception in robotics plays a crucial role in clarifying decision-making processes in sensory processing, enhancing transparency, trust, and interpretability in human-robot interactions, and addressing ethical and safety concerns associated with autonomous systems. By making robotic actions and decisions more understandable and transparent, explainable perception paves the way for safe, reliable, and socially responsible robotics applications in various domains. If you want to learn more about Robotics, we can help you by applying from here to the best institutes for data science course.
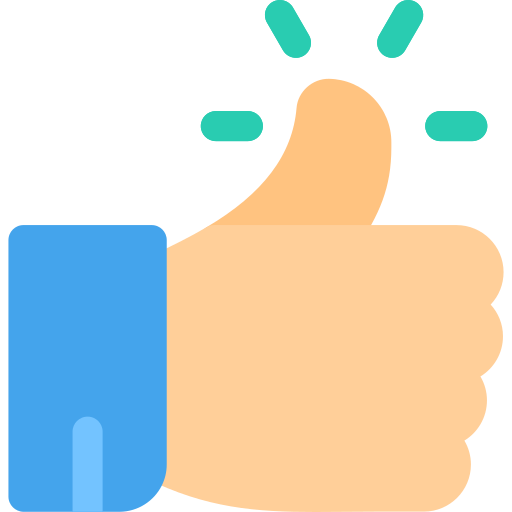
- Industry
- Art
- Causes
- Crafts
- Dance
- Drinks
- Film
- Fitness
- Food
- Games
- Gardening
- Health
- Home
- Literature
- Music
- Networking
- Other
- Party
- Religion
- Shopping
- Sports
- Theater
- Wellness
- News