Data Science pitfalls: Steering clear of common mistakes for success
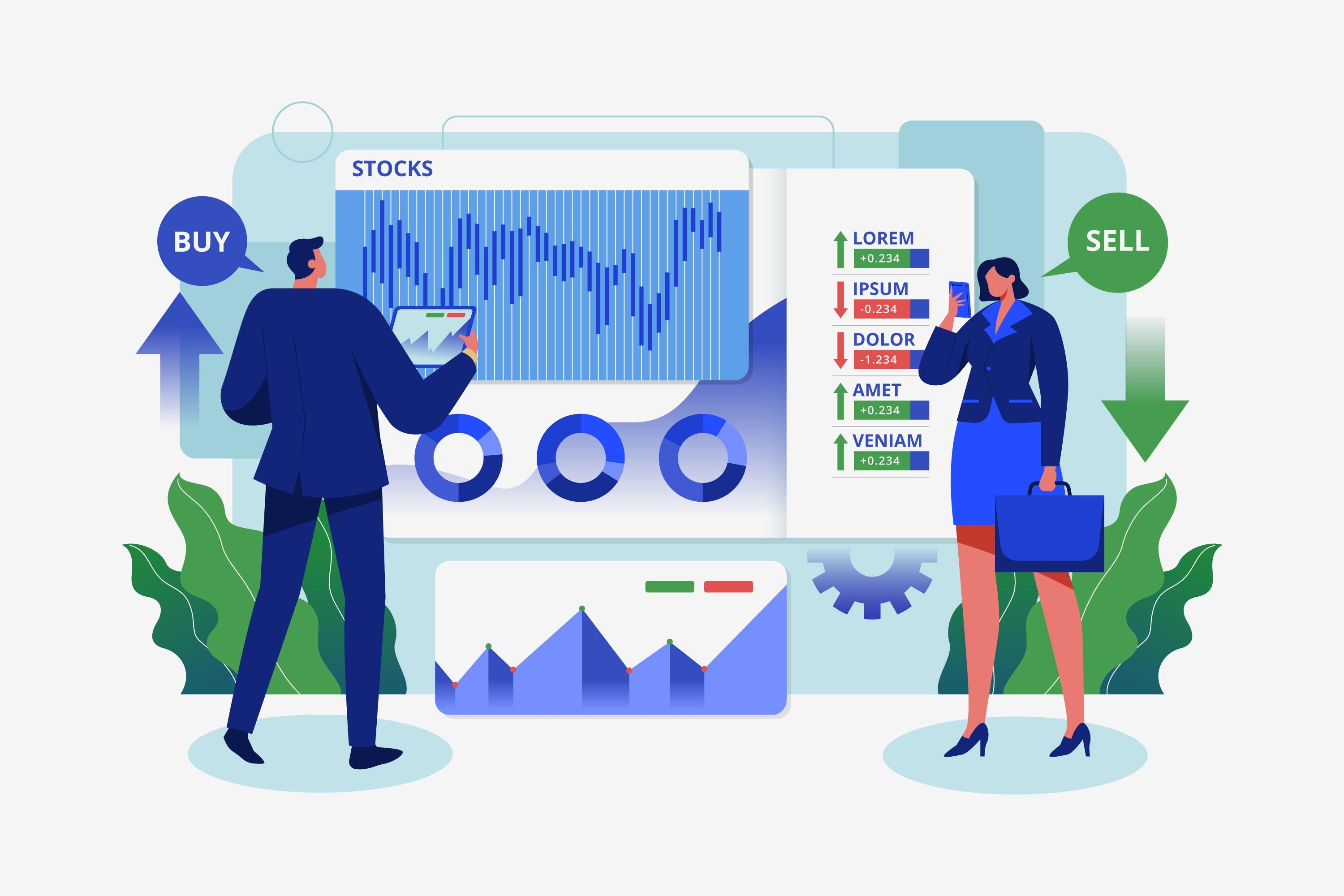
Data Science has emerged as a transformative field, revolutionizing industries across the globe. Showing a more than 650% rise, Data Science is impacting the different industries. By harnessing the power of data, organizations can gain valuable insights and make informed decisions.
However, while the potential of Data Science is immense, it is essential to focus on quality data that can provide accurate insights.
In this article, we will explore some of the most prevalent mistakes in Data Science and provide valuable insights on how to avoid them. When you go for the best Data Science course online you will come to know about it as well.
By understanding and mitigating these challenges, Data Scientists can ensure more accurate analyses, robust models, and ultimately, drive meaningful impact for businesses and society.
Common mistakes to avoid for Data Science
Lack of clearly defined objectives
Without clear goals, your Data Science project may become a ship lost at sea. It's crucial to define what you want to achieve and outline the steps to get there.
Whether it's predicting customer churn or optimizing supply chain logistics, setting clear objectives will guide your analysis and keep you focused on the end goal.
Remember, you're not just playing with data for the sake of it. Your Data Science efforts should align with the needs of the business. Understanding the key challenges faced by your organization and how Data Science can address them will ensure that your work has a meaningful impact.
When you go for Data Science for beginners knowing these mistakes will help you to move ahead further.
Insufficient data preprocessing
Data can be messy. It's like trying to find a needle in a haystack, but the haystack is full of duplicates, errors, and inconsistencies. Before you begin your analysis, it's essential to clean and preprocess the data.
This involves removing outliers, handling missing values, and ensuring data quality. Think of it as decluttering your dataset and creating a solid foundation for accurate results.
Handling missing values
Missing values are like the ghosts of data - they haunt your analysis and can throw off your results. Ignoring missing values or blindly replacing them can lead to biased or incomplete insights.
Instead, use techniques like imputation or consider creating separate models for missing data. By tackling this issue head-on, you can avoid making misguided conclusions.
Dealing with outliers:
Outliers are the rebels of the data world - they refuse to conform to the norm. These extreme values can significantly impact your analysis, skewing results and leading to erroneous conclusions.
Identifying and dealing with outliers through techniques such as truncation or winsorization will help ensure that your models are robust and reliable. Don't let these troublemakers derail your journey!
Remember, Data Science is not just about crunching numbers and building models - it's about extracting meaningful insights that drive real-world impact.
By avoiding common mistakes and applying best practices, you'll be well on your way to becoming a successful data wizard. Happy analyzing!
Overlooking feature selection and engineering:
When it comes to Data Science, it's easy to get caught up in the excitement of building complex models and analyzing massive datasets. But one common mistake that many Data Scientists make is overlooking the importance of feature selection and engineering.
Feature selection is the process of choosing the most relevant variables or features to include in your model. It's important because not all features are created equal – some may be more informative or have a stronger impact on the outcome you're trying to predict.
There are various techniques for feature selection, such as filter methods, wrapper methods, and embedded methods. Each has its advantages and disadvantages, so it's essential to understand which technique is best suited for your specific problem.
Neglecting model evaluation and validation
Another common mistake in Data Science is neglecting proper model evaluation and validation. Building a model is one thing, but ensuring its performance and generalizability is another.
Model evaluation and validation are essential to understand how well your model is performing and how it will perform on unseen data. It helps you assess the reliability and accuracy of your predictions, giving you confidence in deploying your model in real-world scenarios.
Without proper evaluation and validation, you may end up with a model that performs well on your training data but fails to generalize to new data. So, it's crucial to allocate time and resources to this critical step of the Data Science process.
Cross-validation techniques
Cross-validation is a technique to assess the performance of your model on multiple subsets of your data. It helps you estimate how well your model will generalize to new, unseen data and mitigates the risk of overfitting.
There are various cross-validation techniques, such as k-fold cross-validation and stratified cross-validation. These techniques divide your data into training and validation sets, allowing you to evaluate your model's performance on multiple iterations and determine its stability and consistency.
Don't make the mistake of neglecting model evaluation and validation. Properly assessing your model's performance is key to building robust and reliable Data Science solutions.
Disregarding interpretability and explainability
As Data Scientists, we are often obsessed with building the most accurate and powerful models. However, it's essential not to disregard the importance of interpretability and explainability.
Interpretability and explainability refer to the ability to understand and explain how a model makes predictions. While black-box models like deep learning neural networks can achieve impressive performance, they often lack transparency.
Interpretability and explainability are crucial in many real-world applications. They help build trust with stakeholders and end-users, allow for legal and ethical compliance, and enable debugging and error analysis. It's important not to overlook these aspects when developing Data Science solutions.
Failure to continuously update and improve models
Data Science is not a one-time task; it's an ongoing process that requires continuous updates and improvements.
Models can degrade over time due to changing data distributions, evolving user behavior, or shifts in the underlying problem. Therefore, it's crucial to monitor and maintain your models to ensure they remain reliable and effective.
Regularly monitoring your models allows you to detect any performance drop or concept drift, enabling you to take corrective actions promptly. It also helps you identify opportunities for improvement and optimization.
Conclusion
In conclusion, Data Science offers tremendous opportunities for organizations to unlock insights, drive innovation, and make data-driven decisions. However, it is crucial to be aware of the common mistakes that can hamper progress in this field.
By addressing the lack of clearly defined objectives, investing in proper data preprocessing, prioritizing feature selection and engineering, Data Scientists can navigate the challenges and maximize the value of their Data Science endeavors.
If you are someone who wishes to take ahead in their career, enroll now in Pickl.AI Data Science course. Here with the professional certification courses you will be able to learn about all the aspects and there will be no problem throughout.
Enrich your understanding of Data Science and stay ahead in the ever-evolving landscape of Data Science with our dedicated resources.
- Industry
- Art
- Causes
- Crafts
- Dance
- Drinks
- Film
- Fitness
- Food
- الألعاب
- Gardening
- Health
- الرئيسية
- Literature
- Music
- Networking
- أخرى
- Party
- Religion
- Shopping
- Sports
- Theater
- Wellness
- News